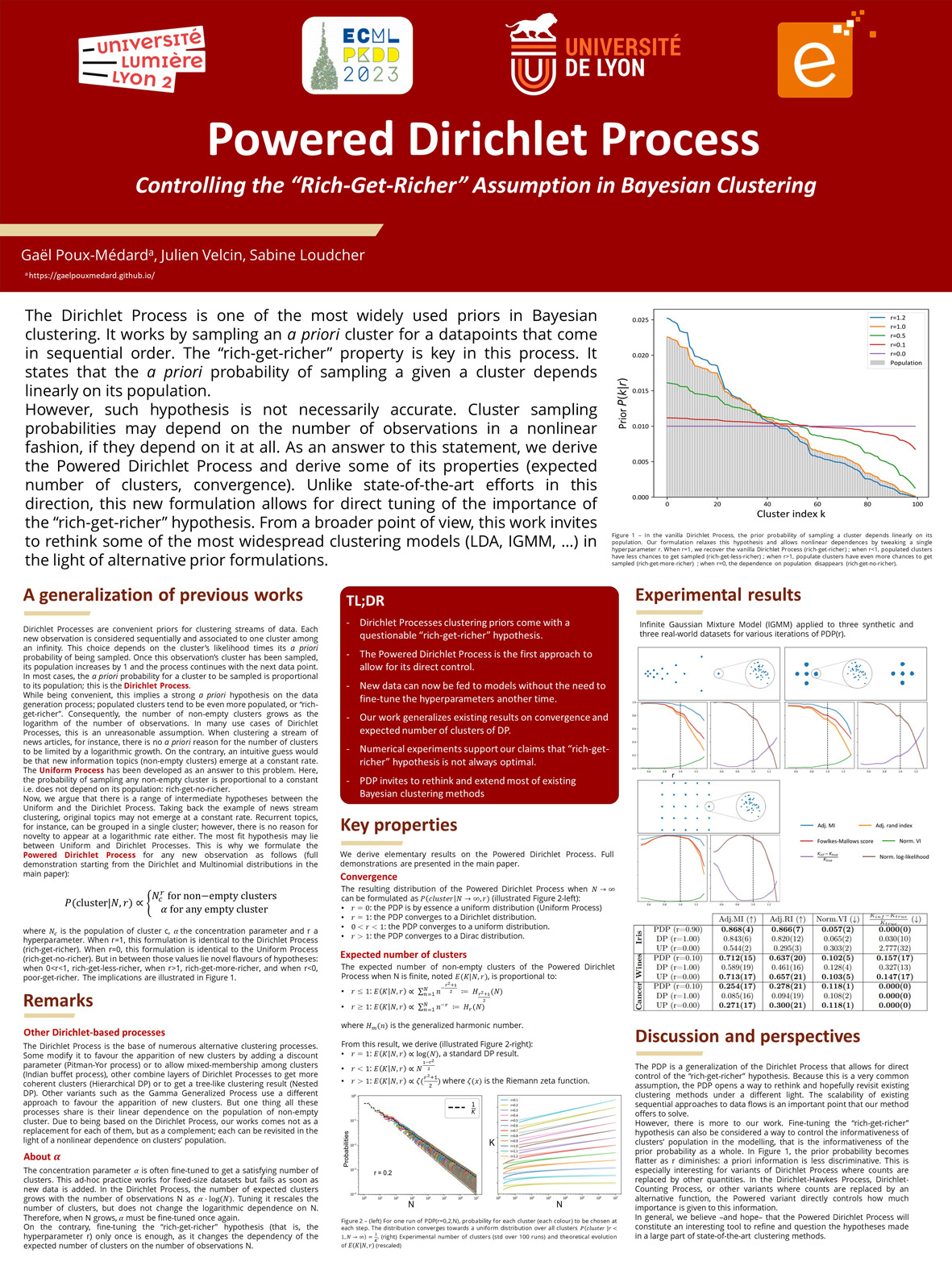
Powered Dirichlet Process - Controlling the ``Rich-Get-Richer'' Assumption in Bayesian Clustering
The Dirichlet process is one of the most widely used priors in Bayesian clustering. This process allows for a nonparametric estimation of the number of clusters when partitioning datasets. The ``rich-get-richer’’ property is a key feature of this process, and transcribes that the a priori probability for a cluster to get selected dependent linearly on its population.
In this paper, we show that such hypothesis is not necessarily optimal. We derive the Powered Dirichlet Process as a generalization of the Dirichlet-Multinomial distribution as an answer to this problem. We then derive some of its fundamental properties (expected number of clusters, convergence). Unlike state-of-the-art efforts in this direction, this new formulation allows for direct control of the importance of the ``rich-get-richer’’ prior. We confront our proposition to several simulated and real-world datasets, and confirm that our formulation allows for significantly better results in both cases.
Slides
Poster
Link to the paper:
Powered Dirichlet Process - Controlling the ``Rich-Get-Richer'' Assumption in Bayesian Clustering